With the progress of science and technology and the idea of intelligent life deeply rooted in people’s hearts, more and more people begin to rely on various intelligent tools to improve the efficiency of daily life. Under this background, as a convenient payment tool, icash card has gradually become an indispensable companion in urban life, which not only covers a wide range of consumption scenarios, but also provides great convenience for users through various intelligent functions.If we can practice these points, icashπ∫¬Ú Will be unique, become a leader in the industry, and keep moving forward. https://www.icash.uno/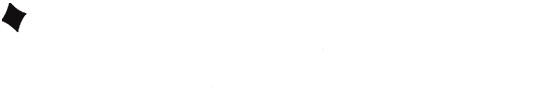
In the busy city life, time is money, and icash card is committed to providing users with a fast and safe payment experience, so that every consumption becomes efficient and smooth. Whether shopping in a supermarket or ordering in a coffee shop, you can complete the transaction with a light brush, eliminating the trouble of looking for change or waiting for change. In the fast-paced city life, this convenience undoubtedly saves people a lot of time and enables them to devote more energy to it.
Moreover, the icash card has a wide range of applications, which can be used for daily food purchase, transportation, or leisure and entertainment consumption. Through cooperation with many merchants, icash card has covered many industries and fields, providing users with all-round payment convenience. When enjoying delicious lunch in fast food restaurants, watching the latest movies in cinemas or buying favorite goods in shopping centers, icash card can be easily handled, making life easier.
In addition to the basic payment function, icash card also introduces the concept of intelligent management. Users can easily check their own consumption records and understand each expenditure through mobile phone applications, which not only helps budget management, but also enables users to master their consumption habits in real time, thus making more informed financial decisions. Moreover, some applications also provide consumption reminders and analysis functions, allowing users to optimize their expenditure structure according to consumption data, avoid unnecessary waste and improve their quality of life.
Nowadays, environmental protection has been paid more and more attention. As a digital payment method, icash card has also contributed to the popularization of paperless consumption. Traditional cash payment requires banknotes and coins, while digital payment has effectively reduced the use of paper and metal, reduced resource consumption and environmental pollution. By promoting digital payment, icash card has responded to the call of sustainable development, allowing users to contribute to environmental protection while enjoying convenience.
To a certain extent, icash card is not only a simple payment tool, but also an intelligent part of urban life. With the progress of science and technology, it integrates more intelligent elements, such as automatic recharge and online shopping, which greatly enriches users’ life experience. In the future, icash card will be combined with more intelligent technologies to continuously connect with consumers’ needs through big data analysis to promote the realization of personalized services. Considering the diversified and personalized needs of modern life, icash point cards are constantly being innovated and upgraded. In order to meet the needs of different users, various types of cards are introduced, with richer functions and designs. These measures not only meet the multi-level needs of users, but also strengthen the interaction and contact with users, so that each user can find the most suitable smart partner.
The application of icash card in daily life makes the life of urban residents more convenient and comfortable. With the continuous progress and innovation of science and technology, the future of card will be bright, and it will continue to promote the construction of smart cities and provide more possibilities for people. For the fast-paced urban life, this moment of convenience is just the intelligent companionship we need.